The realm of artificial intelligence (AI) has undergone a remarkable transformation over the last few decades. Among its many applications, AI filters have emerged as one of the most fascinating and influential. Once a mere concept explored in academic laboratories, today, AI filters are an integral part of our daily lives, shaping the way we perceive and interact with visual content. These filters enhance images and videos in real-time, pushing the boundaries of creativity and communication. As we dive deep into the evolution of AI filters, it’s crucial to understand their early stages, current advancements, and future potential. This journey through time will unveil the intricacies of how AI filtering technology has progressed, revealing not just innovations, but also the significance of ethical considerations that accompany such advancements.
Initially, AI filtering was characterized by basic algorithms that struggled with limitations inherent in computational power and data availability. Early researchers experimented with simple techniques, leading to the development of rudimentary image processing tools. As the field evolved, it required more sophisticated approaches to handle the complexities of visual interpretation. With the introduction of machine learning, AI filters began to take on a new life, transforming the industry as we know it. This advancement not only broadened the scope of applications but also raised important questions about the future trajectory of AI filtering technology.
Early Experiments in AI Filtering
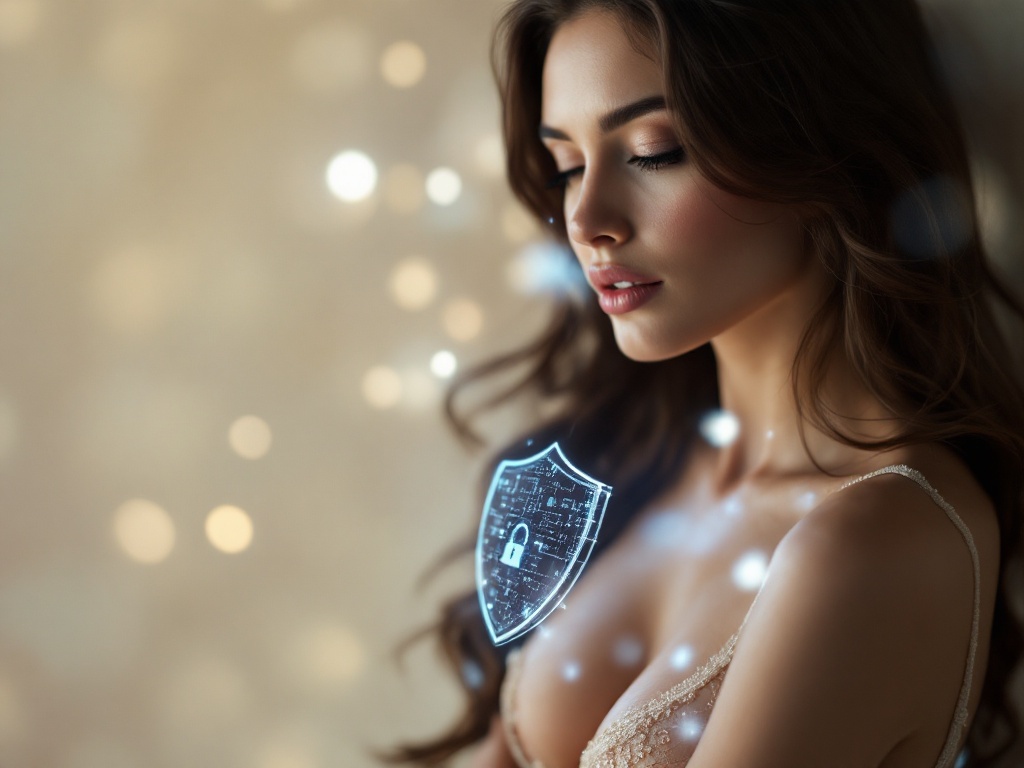
The journey of AI filters began with a series of innovative experiments that laid the groundwork for future developments. Here, we can observe a stark contrast between past limitations and the powerful capabilities of modern filtering solutions. Early algorithmic attempts often involved basic image processing techniques such as edge detection, which focused solely on identifying boundaries within images.
- Edge Detection: The first techniques to identify outlines within images.
- Histogram Equalization: A method aimed at enhancing contrast.
- Template Matching: This involved recognizing specific patterns in images.
While these pioneering tools provided a foundation, they were limited by the computational power available at the time and the lack of substantial datasets. Researchers were able to achieve limited success in creating filters that could intelligently modify images. Problems like blurred images and slow processing speeds were prevalent, leaving much to be desired in the results produced. Nonetheless, these early experiments constituted the initial steps toward a revolutionary evolution in AI filtering.
The Rise of Machine Learning in Filter Development
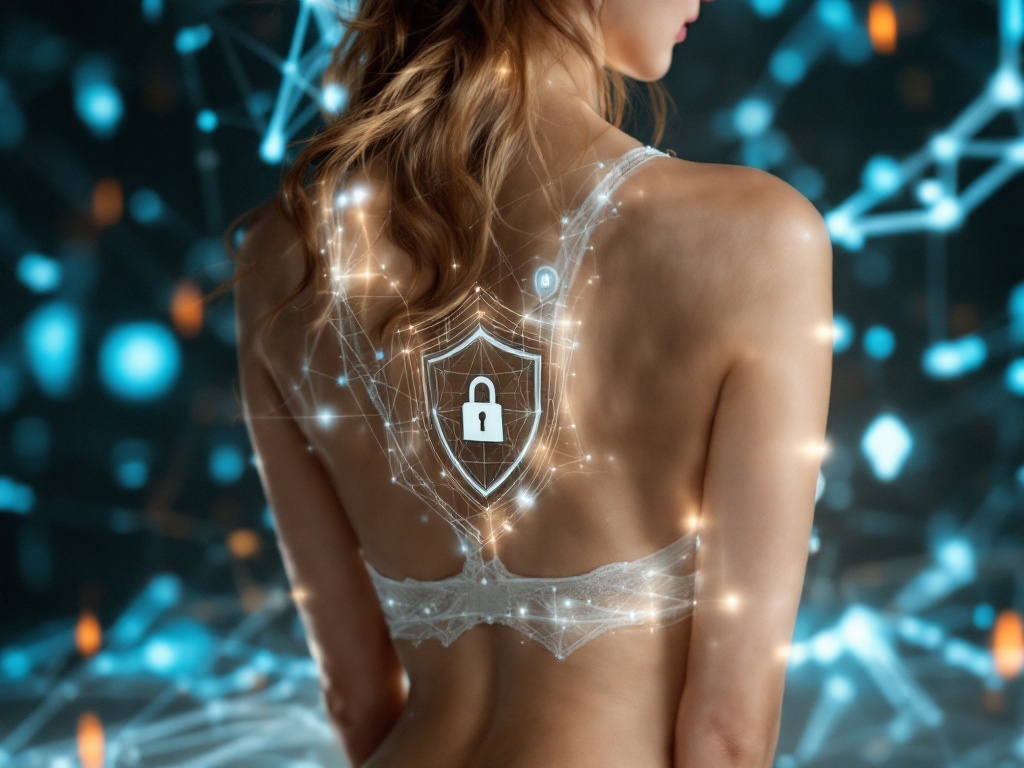
The most significant turning point in the evolution of AI filters came with the rise of machine learning. This transformative approach introduced a paradigm shift in how images and videos could be effectively filtered and enhanced. With the development of more powerful algorithms, particularly neural networks, the performance of AI filters reached unprecedented levels. Machine learning established new frameworks for processing visual data, leading to the creation of filters that could detect and adapt to various visual scenarios.
Neural networks, particularly Convolutional Neural Networks (CNNs), became essential in the evolution of AI filters. These architectures replicate the way the human brain processes visual information, allowing for more nuanced interpretations of images and video content. Key breakthroughs in this realm opened the door to innovative applications across multiple domains, setting the stage for modern solutions.
Today, the integration of AI filters is evident in popular platforms and tools. The following highlights showcase how machine learning has enhanced filtering for images and videos:
- Social Media: Platforms like Instagram and Snapchat utilize AI filters to enhance user-generated content.
- Video Editing Tools: Software applications are increasingly incorporating AI filters to provide users with professional-quality effects.
- Real-Time Gaming: In-game graphics and character designs employ AI to render high-quality visuals instantly.
Feature | Early Filters | Modern AI Filters |
---|---|---|
Processing Speed | Slow and inefficient | Real-time processing |
Accuracy | Limited | Highly precise |
User Interaction | Minimal customization | Extensive user personalization |
Current State of AI Filters
In the contemporary landscape, AI filters have become increasingly sophisticated and widely adopted. Several advanced techniques and tools are transforming the way we interact with visual content. Current AI filtering technologies utilize cutting-edge machine learning algorithms, allowing for enhanced performance and adaptability.
- Real-Time Processing: Many applications offer on-the-fly enhancements, crucial for live-streaming and social media.
- Augmented Reality Filters: Used predominantly in mobile apps, enabling interactive experiences for users.
- Content-Aware Editing: Filters that analyze the context of an image and apply adjustments intelligently.
Nevertheless, the rise of AI filters also raises various ethical considerations. The implications of embedding filters into everyday technology include concerns regarding privacy, misinformation, and the potential misuse of capabilities to create misleading content such as deepfakes. The industry is increasingly grappling with these ethical ramifications, paving the way for possible regulations to ensure responsible use of filtering technologies.
The Future of AI Filters
As we look ahead, the future of AI filtering technologies appears brighter and more complex. Continuous innovations are expected to emerge, focusing on enhanced accuracy and improved efficiency in processing. AI filters will likely take advantage of augmented reality (AR) and virtual reality (VR) advancements, opening new avenues for creativity and engagement.
- Hyper-Realistic Filters: Future filters may provide an even more authentic appearance, mimicking real-life more closely than ever.
- Cross-Technology Integration: Collaboration with IoT devices could lead to more personalized user experiences.
- Healthcare Applications: Utilizing AI filters for medical imaging analysis may allow for better diagnostics and patient outcomes.
Moreover, as AI filters integrate with other emerging technologies, we could witness revolutionary use cases across various sectors, including healthcare, entertainment, and security. The sophistication of these tools will enhance their applicability, propelling the evolution forward and broadening their potential impact on society.
Conclusion
The journey of AI filters from early experiments to modern solutions illustrates a captivating evolution filled with challenges and breakthroughs. Understanding this transformation is essential not just for industry professionals and enthusiasts, but also for users who interact with these technologies daily. As AI filters continue to advance, embracing the associated ethical considerations will be crucial to ensure their responsible and beneficial use in society. The future holds exciting possibilities, and by staying informed, we can appreciate the profound impact of AI filtering technologies.
Frequently Asked Questions
- What are AI filters? AI filters are algorithms that process and modify content, such as images and videos, using techniques that mimic human perception and analysis.
- How have AI filters changed over the years? AI filters have evolved from rudimentary algorithms to sophisticated systems powered by machine learning and neural networks, allowing for real-time processing and more complex effects.
- What are some common applications of AI filters today? Common applications include social media enhancements, video streaming, and image editing tools that provide real-time effects and modifications.
- What ethical concerns exist regarding AI filters? Ethical concerns include privacy violations, the potential creation of misleading content (such as deepfakes), and the societal impacts of manipulated imagery.
- What future trends can we expect in AI filtering technology? Innovations may include better accuracy, integration with AR/VR, and more diverse applications in sectors like healthcare and entertainment.