The rapidly developing world of technology demonstrates that machine learning is transformational, particularly in how businesses function, as in the case of supply chain management. In recent years, competition and new requirements have intensified, forcing firms to adopt cutting-edge technologies. By implementing machine learning, companies are now able to analyze enormous amounts of data and instantly convert it into logistical improvements. This technological innovation efficiently aids almost every aspect, from inventory management to demand prediction, allowing prompt responses to market fluctuations. Envision a situation where allocating resources is as effortless as achieving productivity; such a transformation is certainly possible. As a matter of fact, there are thousands of firms innovating effectively, and it is hardly surprising.
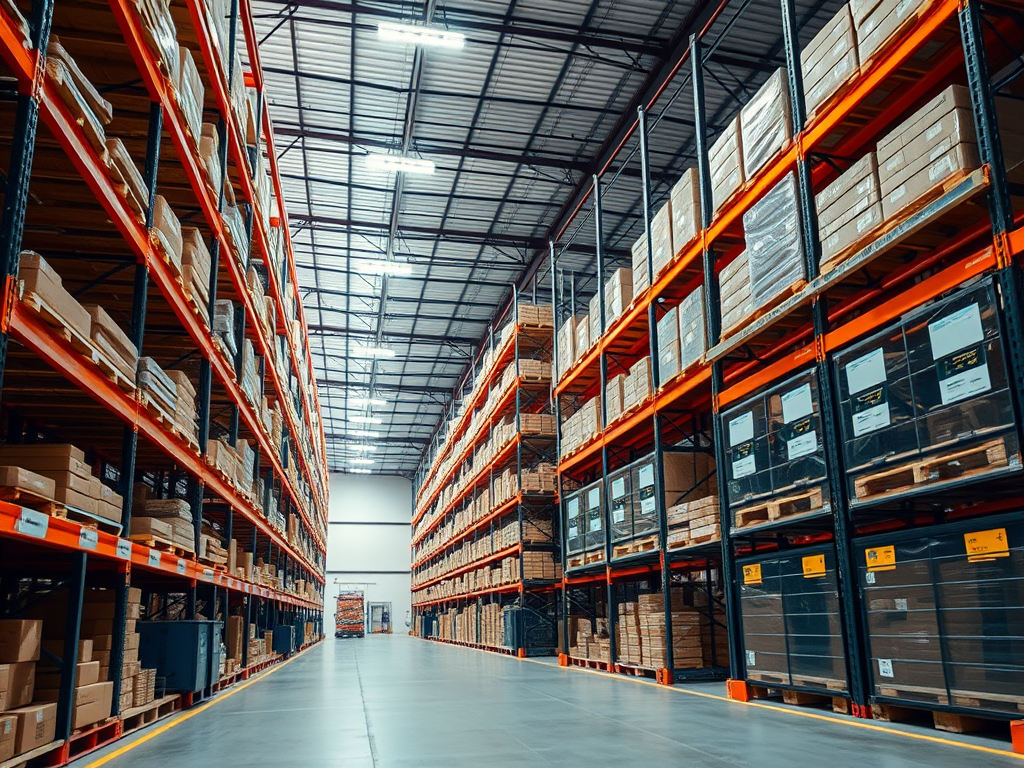
Key Applications of Machine Learning in Supply Chains
The use of machine learning in supply chain management is steadily developing and improving the quality of decision-making. The applications of these systems range from predictive and prescriptive analytics to advanced forms of automation. Systematic demand forecasting enables companies to predict consumer demand with astonishing accuracy. Automated reorder systems simplify inventory management by stocking up on items without excessive expenditure on storage space. Supplier selection and inventory management are equally effortless, as suppliers are chosen based on their previously assessed performance records along with the calculated risks. Even the optimization of delivery routes can take advantage of machine learning, as timely deliveries can be achieved through traffic pattern analysis and consideration of unforeseen events.
Demand Forecasting
Our expectations for anticipating the demand for services and products have changed drastically due to the incorporation of machine learning algorithms. Historically, the common practice for estimating future demand was through intuitive forecasting techniques; however, this frequently created gaps and misinformation. On the other hand, machine learning offers automation that analyzes external circumstances, sales history, seasonal trends, etc., and accurately anticipates sales figures. This not only maximizes business efficiency by enhancing cash flow through avoiding stock-outs and excess inventory, but also improves overall business performance. Businesses that adopt the new forecasting techniques not only realize greater profit margins but also achieve increased customer satisfaction.
Inventory Management
Desktop tracking methods are swiftly giving way to computerized inventory systems. Using machine learning analytics, organizations can now determine the best time to restock a product by using sales and inventory movement as restock triggers. Identified stock optimization increases space efficiency while lowering holding costs. The removal of manual tracking errors improves a firm’s responsiveness to market fluctuations. Automated tracking of this nature ensures that products are only available when needed, allowing customer satisfaction to soar. In addition, a recent study indicated that companies equipped with artificial intelligence-driven inventory control systems reduced their wastage to nearly thirty percent.
Application | Benefits |
---|---|
Demand Forecasting | Increased accuracy in sales predictions |
Inventory Management | Reduced holding costs and waste |
Supplier Selection | Enhanced vendor evaluation and risk management |
Route Optimization | Improved delivery times and reduced costs |
Application Benefits Application Benefits Demand Forecasting Increased accuracy in sales predictions Demand Forecasting Increased accuracy in sales predictions Inventory Management Reduced holding costs and waste Inventory Management Reduced holding costs and waste Supplier Selection Enhanced vendor evaluation and risk management Supplier Selection Enhanced vendor evaluation and risk management Route Optimization Improved delivery times and reduced costs Route Optimization Improved delivery times and reduced costs
Benefits of Incorporating Machine Learning in Supply Chains
Applying machine learning technology has a significant impact on supply chains in both positive and negative ways. These include the automation of tasks that were previously manual, which increases operational effectiveness by saving time on decision making and minimizing the strategic workload in an organization. Apart from this, there are savings as a result of the elimination of several operational expenses. Additionally, reducing money spent on overstocking inventory, wasteful logistics, and inefficient inventory level optimization may help decrease overall waste. Stakeholders are more satisfied due to the machine learning implementation increasing accuracy in order fulfillment and timely deliveries, which in turn raises customer satisfaction. Companies that adopt this technology become more competitively positioned within the industry.
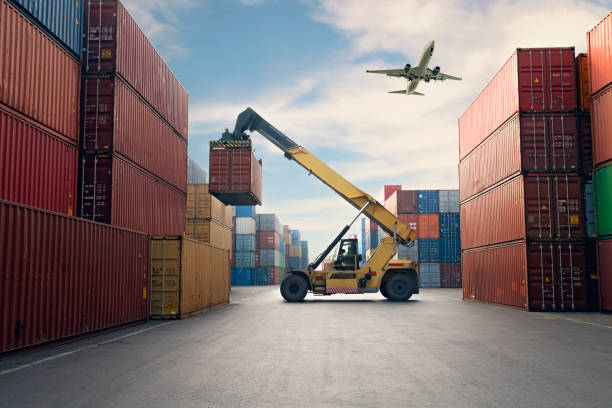
Challenges in Implementing Machine Learning in Supply Chains
Despite the clear benefits, implementing machine learning comes with its challenges. Data quality is one of the more pertinent challenges because ML algorithms require well-defined and high-quality datasets. In the absence of adequate data, even powerful algorithms produce poor results; therefore, strong data governance policies in an organization are critical. Also, some individuals may be set in their ways because of the fear of losing their jobs or being overwhelmed by technology. Addressing these cultural barriers requires thoughtful change management and significant training. Additionally, the need to implement machine learning features into various systems generates interoperability issues, which may necessitate substantial spending on new system software.
Conclusion
It is a known fact that machine learning is more effective in improving supply chain management for the better, and it is also affecting business operations. The current market leaders who have adopted the technology are achieving higher operational efficiency, lower costs, and improved customer satisfaction. The future with advancements in machine learning seems promising, as there will be more disruptive innovations that can further streamline supply chains. Companies need to adapt quickly to these changes to stay competitive, and this requires investment in talent and technologies. Consequently, they will be able to create more durable, efficient, and modern supply chain systems.
Frequently Asked Questions
In what ways does machine learning enhance demand forecasting? Machine learning enhances demand forecasting by using large datasets to detect patterns and trends that aid in forecasting demand with greater accuracy.
What part of the processes is automated with the use of machine learning in inventory control? ML algorithms automate the control of inventory by predicting the optimal times for reordering products and reducing the chances of overstocking.
Can machine learning help minimize supply chain spending? Indeed, machine learning can help reduce spending by optimizing inventory and logistics costing processes.
Why do some people fail at utilizing machine learning technology on supply chain processes? The common hurdles include poor quality data, unwillingness to change, and lack of compatibility with current systems.
Can every business in supply chains apply machine learning? Although many businesses can benefit from machine learning, its application depends on the readiness of the industry, data, and organizational structure.